
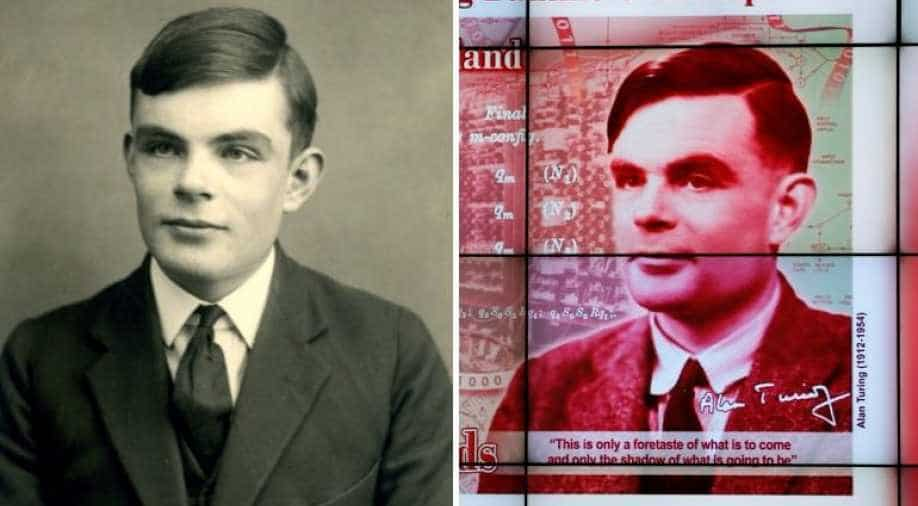
Our Bayesian formulation extends the work on semantic parsing (P. In, we propose a symbolic-based approach to answer questions about images, where we combine a 'soft' perception with a symbolic reasoning.
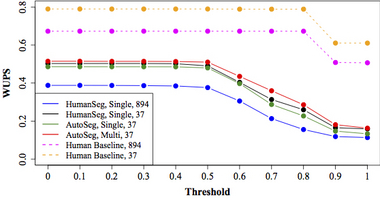
We enumerate the challenges that holistic learners have to face with, and discuss advantages of the question answering task over other high cognitive problems, most prominently scalable annotation effort and automatic benchmarking tools.
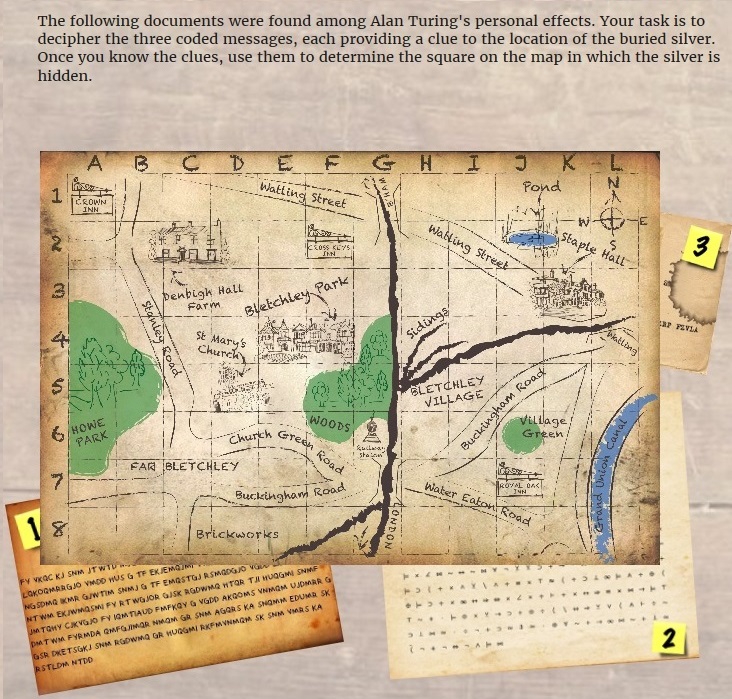
In and, we provide a wider view on question answering based on images task. Moreover, we also explore different ways of benchmarking the machines on this complex and ambiguous task. In this line of research, our primary focus lies on building machines that answer questions about images. This trend has allowed the community to progress towards more challenging and open-ended tasks and refueled the hope at achieving the old AI dream of building machines that could pass a turing test in open domains. As language and visual understanding by machines progresses rapidly, we are observing an increasing interest in holistic architectures that tightly interlink both modalities in a joint learning and inference process.
